Machine Learning
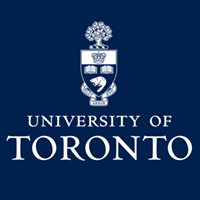
Timeline
-
March 10, 2020Experience start
-
March 13, 2020Project Scope Meeting
-
March 14, 20202nd Touch Point
-
April 4, 2020Project Presentation
-
April 14, 2020Experience end
Timeline
-
March 10, 2020Experience start
-
March 13, 2020Project Scope Meeting
Meeting between students and company to confirm: project scope, communication styles, and important dates.
-
March 14, 20202nd Touch Point
Evaluating initial findings, clarifying possible doubts, and revisiting plan for the remainder of the project.
-
April 4, 2020Project Presentation
Presenting the project to the company
-
April 14, 2020Experience end
Categories
Communications Operations Project management Marketing strategySkills
business analytics business consulting data analysis machine learning artificial intelligenceThroughout the course students under instructor supervision will create machine learning solutions to enable your organization to predict future events of interest.
The final project deliverables will include:
- The POC software created to solve the machine learning problem - it is not a production ready solution
- Recommendation report on how to use the model
- A final presentation
Project timeline
-
March 10, 2020Experience start
-
March 13, 2020Project Scope Meeting
-
March 14, 20202nd Touch Point
-
April 4, 2020Project Presentation
-
April 14, 2020Experience end
Timeline
-
March 10, 2020Experience start
-
March 13, 2020Project Scope Meeting
Meeting between students and company to confirm: project scope, communication styles, and important dates.
-
March 14, 20202nd Touch Point
Evaluating initial findings, clarifying possible doubts, and revisiting plan for the remainder of the project.
-
April 4, 2020Project Presentation
Presenting the project to the company
-
April 14, 2020Experience end
Project Examples
Machine learning is a field in computer science that uses statistical techniques to give computer systems the ability to learn from data without being explicitly programmed. Developing effective machine learning is often challenging; finding patterns is difficult and there is often not enough training data available.
Project examples include, but are not limited to:
- Predicting future events of interest: For instance, in marketing, machine learning enables businesses to predict customer intents, including purchasing a product or terminating their service contract. In area of predictive maintenance, machine learning can predict health status of different equipments and help business take proactive action towards maintaining operating at low cost.
- Spotting anomalies in data sets: Detecting fraud is a use case in point of sales and banking transactions
Companies must answer the following questions to submit a match request to this experience:
Ensure you have "enough real data" and be ready to share data with the instructor 1-2 week before the project starts. This is crucial in success of the project.
Be available for a quick phone call with the instructor to initiate your relationship and confirm your scope is an appropriate fit for the course.
Provide a dedicated contact who is available to answer periodic emails or phone calls over the duration of the project to address students' questions.
Provide an opportunity to students to present their work and receive feedback
Be available for at least 2 follow up meetings (phone call, preferably in-person) with the students to monitor the progress, clarify doubts, and answer questions.
Timeline
-
March 10, 2020Experience start
-
March 13, 2020Project Scope Meeting
-
March 14, 20202nd Touch Point
-
April 4, 2020Project Presentation
-
April 14, 2020Experience end
Timeline
-
March 10, 2020Experience start
-
March 13, 2020Project Scope Meeting
Meeting between students and company to confirm: project scope, communication styles, and important dates.
-
March 14, 20202nd Touch Point
Evaluating initial findings, clarifying possible doubts, and revisiting plan for the remainder of the project.
-
April 4, 2020Project Presentation
Presenting the project to the company
-
April 14, 2020Experience end